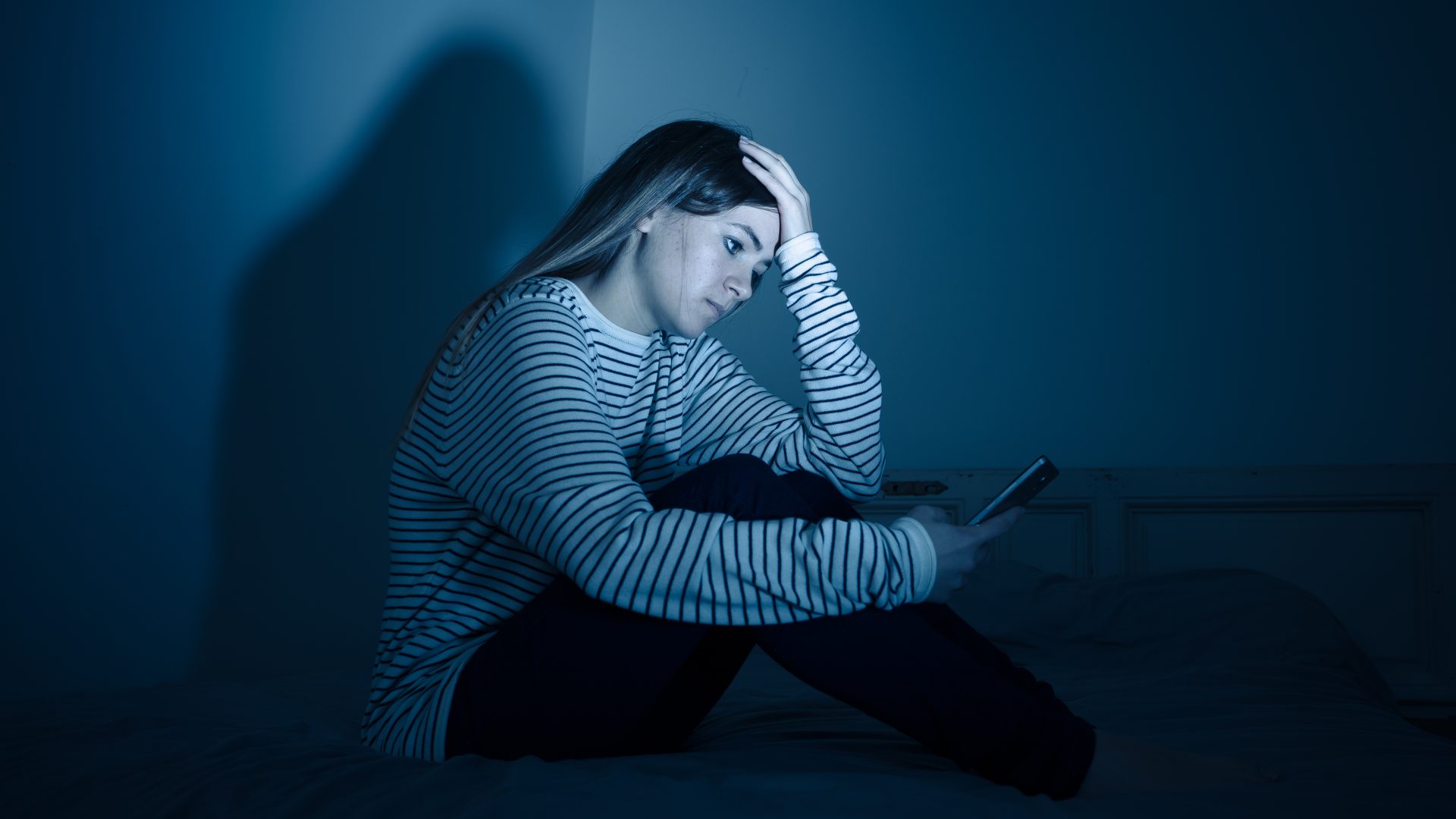
Generative AI: the new frontier for gendered disinformation?
Concerns about the impact of generative AI on elections are increasing as the tools grow in sophistication and become more accessible. This raises multiple concerns about the potential impact on electoral misinformation and disinformation (which we discuss in our new report, Synthetic Politics). One of these threats to democracy that needs more attention is the growing risk of online gendered disinformation. This year has seen political division over reproductive rights, gender-affirming care, and drag queen performances, and where political divisions lie, disinformation campaigns follow and exploit.
Gendered disinformation is a form of online violence which weaponises gendered stereotypes to abuse, demonise and undermine people in public life, disproportionately targeting Black, LGBT+ and other minoritised women. Because gendered disinformation often focuses on sexualising its targets, the new ease of image and video generation is particularly concerning, from enabling the fabrication of intimate images of sexual activity to other private images of people. Faked pornographic images are of course an old, pre-gen AI tactic. But generative AI tools means this creation is easier, and being able to instantaneously create convincing images and video adds a new and powerful string to the bow of bad actors.
The threat of this is not just that people might believe false things about women in public life. In a shock-driven online environment, even deepfakes that no-one believes are real will spread like wildfire. This is how information pollution works – not just that there are untruths out there, but that those untruths compete for space with everything else, appearing as automated search completions, or as suggested hashtags, or as recommended videos. And when those untruths are not just untrue but violent, a polluted environment becomes a toxic and unsafe environment for individuals and for entire communities. This in turn has serious ramifications for who is able to participate in public life.
Action is being taken – with AI companies banning these usages and social media banning the sharing of this kind of content, as well as collaborating on further commitments to tackle harms from deceptive AI-generated content in elections. Policymakers have acted too: from the criminalisation of sharing non-consensual intimate images, including deepfakes, through UK the Online Safety Act and an EU Directive on violence against women, and there are calls for the creation of such deepfake pornography to be criminalised. The EU AI Act also requires producers of generative AI tools to disclose when the products of the tools are deepfakes.
But disclosing deepfakes will not tackle the violence inherent in nonconsensual intimate image sharing – while relying on the criminal law is not going to be able to effectively tackle these risks at scale. And despite bans, gendered violence exploits any loopholes possible. The recently shared explicit deepfake images of Taylor Swift were created by an online community working out how to get around technical safeguards. Moreover, there are many – and lucrative – generative AI tools created specifically for the purpose of deepfake pornography.
In the immediate term, with elections ramping up, there need to be clear channels for communication and collaboration between social media platforms, civil society and candidates, so that escalating threats can be recognised and amplification mitigated. Political parties should also ensure their candidates have appropriate digital safety and psychological support.
But in the longer term, these post-hoc responses to attacks are not sufficient. These risks aren’t going to be able to be properly addressed until a gender lens is applied throughout the process of technological development. This should go from informing what tools are designed in the first place, to shaping risk assessments and testing processes – and will require technologies to be genuinely co-created with people who are marginalized on the basis of gender.